|
|
Object recognition is fundamental in the lives of most animals. The authors review research comparing object recognition
in pigeons and humans. One series of studies investigated recognition of previously learned objects seen in novel depth
rotations, including the influence of a single distinctive object part and whether the novel view was close to two or only
one of the training views. Another series of studies investigated whether recognition of directly viewed objects differs from
recognition of objects viewed in pictures. The final series of studies investigated the role of motion in object recognition.
The authors review similarities and differences in object recognition between humans and pigeons. They also discuss future
directions for comparative investigations of object recognition. |
|
Introduction
For most creatures, successful interactions with the world
require the ability to rapidly detect, recognize, and respond
to numerous objects, both animate and inanimate. For example,
rapid and accurate recognition of a mate, a predator,
an edible object, or a navigational landmark would all
be beneficial for survival. Indeed, the very fact that almost
all complex organisms have evolved sophisticated sensory
systems to determine “what’s out there” underscores the importance
of detecting external stimuli and recognizing what
they are. Object recognition thus goes beyond simple detection
and requires cognitive processes to recognize, interpret,
and appropriately respond to objects. Because survival can
depend on the ability to perform these operations quickly
and accurately, it follows that the evolution of cognitive processes
necessary for object recognition should be widespread
throughout the animal kingdom. It does not necessarily follow,
however, that the nature of these processes is the same
across all species. Just as sensory systems have evolved with
both common and specialized features across species, we
might expect to see both general and specialized cognitive
processes for interpreting the information detected by sensory
systems. Thus, object recognition is fruitful ground for
investigations of comparative cognition.
It takes only a few moments of thought to realize that the
ability to recognize objects is not only widespread across
species, but it is also a fundamental part of almost every
waking moment of our lives. In fact, only when we experience
problems in recognizing objects do we even notice that
we are continually processing stimuli to determine what is
out there. But just because object recognition is routine and
fundamental does not mean that it is simple. In fact, visual
object recognition is an extremely complex ability, due to
the fact we must construct a three dimensional (3-D) world
from two dimensional (2-D) input. That is, the 3-D objects
in the world are first registered on the retinas at the back of
each eye, which, for practical purposes, are 2-D. The 2-D information from each eye is combined to form the 3-D representation
of the world. Moreover, we must parse complex
patterns of light into separate objects, and often we must infer
the existence of whole objects from incomplete information.
Thus, as illustrated in Demonstration 1, what we ultimately
“see” goes beyond the pattern of light that reaches our eyes.
Another complexity faced by any object recognition system
is that the information reaching our eyes changes across
viewpoints. In fact, the 2-D outlines of the same object seen from different views are sometimes less similar than the
outlines of different objects (e.g., consider the 2-D silhouettes
formed by the image of a horse from the front vs. the
side view, compared to the 2-D silhouettes formed from the
side views of a horse vs. a donkey). Thus, it is no simple
task to be able to both discriminate between similar-looking
objects and to recognize different-looking views of the
same object. How visual cognitive systems accomplish these
feats, and with the speed needed to interact successfully with
the world, has been the focus of considerable research and
theory in human cognition (e.g., Biederman, 1987; Bülthoff
& Edelman, 1992; Tarr & Bülthoff, 1998), and more recently
in animal cognition (e.g., Friedman, Spetch, & Ferrey, 2005;
Kirkpatrick, 2001; Logothetis, Pauls, Bülthoff, & Poggio,
1994; Peissig, Young, Wasserman & Biederman, 2000;
Spetch, Friedman, & Reid, 2001).
Our review will focus on a series of recent studies conducted
in our laboratories that were aimed at comparing the
cognitive processes that underlie recognition of 3-D objects
in humans with the processes used by pigeons. Theses species
provide an interesting comparison because pigeons,
like humans, are highly visual creatures, but they differ substantially
from humans both in their visual experiences and
in the neuroanatomy of their visual system (see Zeigler &
Bischof, 1993; Husband & Shimizu, 2001). For example,
birds, through flight, may require a different set of processes
for rapid comprehension of the 3-D world than do humans.
Pigeons also have two fovea-like specialized retinal areas
that are each similar to the human fovea. One appears to
be specialized for near frontal vision and presumably facilitates
detection and selection of grain, and one appears to
serve more distant monocular lateral vision, and may allow
constant monitoring of predators (see Blough, 2001). Our
studies, and other research we will review, suggest that there
are both interesting similarities and important differences
between the cognitive processes underlying the bird’s eye
view of the world and those that underlie our own.
Because the direction of theoretical influence in object
recognition literature has been from human work to comparative
studies, our review will start with a brief overview
of theories of object recognition in humans. We will then review
comparative studies of object recognition, with a focus
on studies of pigeons and humans that concern three select
aspects of object recognition: viewpoint dependence, transfer
between objects and pictures, and the role of dynamic
cues. We will suggest that processes of object recognition
in pigeons share several similarities to those that underlie
human object recognition, but that there also appear to be
some interesting differences between species. We end by
noting that comparative studies of object recognition are at
an early stage of both empirical and theoretical development
and suggest that an ecological approach may provide an excellent
complement to current research programs.
Theories of Human Object Recognition
One of the most intriguing aspects of object recognition is
our ability to identify objects across changes in viewpoint. In
particular, depth rotations of an object can drastically change
the 2-D information that reaches our eyes. Explaining how
we recognize an object despite these changes has been a
major theoretical challenge. Two general classes of theories
have been proposed to explain how humans recognize objects
when seen from novel views. The classes differ principally
in terms of how the shapes, features, and structure of
objects are represented, and what processes are involved in
object recognition.
In one class of theories, shape representations are object-based,
insofar as they consist of “structural descriptions”
of the 3-D properties of the objects. Because an object’s
structure is represented, there is no one view of it that is
“privileged” (e.g., more accessible or recognizable) in any
sense. For example, the recognition-by-components theory
(Biederman, 1987) and its more recent version, the geonstructural-
description (GSD) theory (Biederman & Gerhardstein,
1993), assume that representations of objects consist
of simple, volumetric parts (called “geons”) and the spatial
relations among them. Both the geons themselves and their
spatial arrangement are thought to be important for recognition.
According to these theories, recognition of an object
should be invariant across changes in view as long as
certain conditions are met, namely that: 1) the object can
be decomposed into geons, 2) the arrangement of the geons
forms a distinct structural description that differs from other
arrangements, and 3) changes in the view of the object do
not change the structural description (as would be the case,
for example, if a particular part was visible only from certain
views). Thus, object-based theories predict that all views of
an object that meet these three criteria should be recognized
with approximately the same speed and accuracy.
The second class of theories assumes that objects are encoded
in memory in the poses in which they are seen by
viewers, and are thus called “view-based” theories (e.g., Bülthoff & Edelman, 1992; Edelman, 1999; Tarr, 1995; Tarr
& Pinker, 1989). In the earliest version of this class (Tarr,
1995; Tarr & Pinker, 1989), it was assumed that because objects
can be seen from many views, the representation of an
object emerges as a collection of stored views. Each stored
view reflects the specific metric properties (appearance) of
the object as it looks from that view. Thus, the ability to
recognize the object when seen from a novel view requires
a mechanism that matches the current percept to one of the
stored views, except in cases where recognition can be based
on the presence of a distinctive, diagnostic feature. For example,
if one can see the trunk of an elephant, it is probably not necessary to see much else in order to identify the elephant
as such. In the absence of such a diagnostic feature,
however, the view-based theory predicts that speed or accuracy
in recognizing an object will decrease as a function of
the rotational distance between a given novel view and the
nearest stored view.
In many situations, object- and view-based approaches to
understanding object recognition make the same predictions.
Specifically, when an object contains a single distinctive geon that can serve as a diagnostic feature, both view-based
and object-based approaches predict that the speed and accuracy
of recognition will be viewpoint invariant. Conversely,
whenever one or more of the three conditions for viewpoint
invariance stipulated by object-based theories is not met,
then both classes of theories predict that performance will
exhibit viewpoint dependence. Because the predictions that
are common to both classes of theories have been supported
in studies of human object recognition, the challenge for researchers
has been to identify situations that test differential
predictions of the two classes of theories.

Figure 1. Paperclip-like object, and objects containing one,
three or five added geons. From Tarr et al., 1997. |
|
Tarr, Bülthoff, Zabinski, and Blanz (1997) identified and
tested one such situation. Specifically, they noted that if
objects consist of unique arrangements of multiple distinguishable
geons that can be seen from all views, then they
meet the conditions for viewpoint invariance according to
the object-based approach. However, if the objects also do
not contain a single unique part that can serve as a diagnostic
feature, view-based theories predict that object recognition
should be viewpoint dependent. To test these differing predictions,
Tarr et al. (1997) examined peoples’ recognition of
depth-rotated objects in four conditions. In one condition,
the stimuli consisted of paperclip-like objects with no added
geons (Figure 1, left object). Both classes of theories predicted
viewpoint dependence for these objects. In the second
condition, one distinctive geon was added to each object,
and the added geon differed across objects making it a
diagnostic feature (Figure 1, second object from left). Thus,
both classes of theories predicted that recognition should
be viewpoint invariant in this condition. In the remaining
conditions, either 3 or 5 distinctive parts were added to the
objects (Figure 1, rightmost two objects). These new parts
were arranged in unique ways for each object, thus fulfilling
the three conditions of the object-based theories, but the
specific geons used to create the objects were not unique
to each object. Thus, for the multi-part objects, each object
had a unique structural description, but no particular geon
could serve as a diagnostic feature for any of the objects. In
this case, object-based theories predict viewpoint invariance
whereas view-based theories predict viewpoint dependence.
Consistent with the predictions of view-based theories, Tarr et al. (1997) found that people showed strong viewpoint
dependence in the multi-part conditions, both in a naming task, in which people learned one-syllable names for each of
several novel objects and then identified them in either the
learned view or new views, and in a same-different task in
which people simply had to detect whether two views represented
the same object.
Although there is now quite a bit of evidence favoring
view-based object recognition for humans, the view-based
approach has recently undergone a challenge to both its representational
and process assumptions. In its original instantiation
(Tarr, 1995; Tarr & Pinker, 1989; see also Shepard
& Metzler, 1971), a 2-D representation of a novel view was
“normalized” (i.e., transformed) until it matched one of a set
of 2-D “snapshot-like” stored representations. We will thus
refer to this as the normalization approach. The increase in
time and decrease in accuracy that was typically observed
as a function of rotational distance between the novel and
stored views was hypothesized to arise from the length of
the transformation process itself. As originally conceived
(Shepard & Metzler, 1971; Metzler & Shepard, 1974; Tarr
& Pinker, 1989), this process involved a kind of “mental
rotation” that transformed the novel percept until a match
was achieved (or not) to a stored view. Thus, the larger the
rotational distance between the two the longer (and less accurate)
would be the predicted response.
In recent years, a new view-based approach to object
recognition has been developed that has as its basis a more
sophisticated representational scheme as well as a computational
recognition mechanism that appears to explain
a broader range of phenomena than the normalization approach
(Broomhead & Lowe, 1988; Bülthoff & Edelman,
1992; Edelman, 1999; Edelman & Bülthoff, 1992; Edelman,
Bülthoff, & Bülthoff, 1999). In this view-combination approach,
some novel views of familiar shapes can benefit
during recognition from their similarity to more than one
learned view. Thus, this approach is a type of view-based
approach, but it is more robust because it can accommodate
situations in which performance appears to be view-dependent
and others in which it appears to be view-invariant.
The two view-based approaches can be distinguished by
their predictions for a situation in which participants are
trained with more than one view of a given object. Consider, for example, participants who are trained with two views
of an object that differ from each other by a 30° rotation in
depth (we will arbitrarily call these the 0° and 30° views).
They may be tested with novel views that are either interpolated
within the shortest distance between the trained views
(e.g., 15°) or are extrapolated outside of this training range
(e.g., 45°). On such tests, participants show more accurate
recognition of the interpolated novel views than they
do for the extrapolated novel views (Bülthoff & Edelman,
1992).
This finding is troublesome for normalization accounts of
object recognition, because these accounts predict that recognition
performance for both interpolated and extrapolated
novel views should be equal and inferior to performance on
the learned views (Tarr & Pinker, 1989; Tarr, 1995). Again,
this is because it is assumed that objects are represented as a
number of “exemplar” views (e.g., the training views), and
that novel views are recognized by transforming them until
they are aligned with the nearest stored exemplar (e.g., Ullman,
1989). Thus, recognition performance is predicted to
be a declining function of distance between a novel view and
its nearest stored exemplar, and the interpolated and extrapolated
conditions are usually equated on this factor. Indeed,
even when the interpolated view is equidistant to two different
training views it is still assumed to be normalized to only
one of them on each trial.
As noted above, in contrast to the normalization approach,
view combination approaches permit some novel views to
benefit from their similarity to more than one learned view.
For example, Edelman (1999; see also Broomhead & Lowe,
1988; Poggio & Edelman, 1990) assumes that objects are
represented as points in a multidimensional shape space
spanned by similarities to a small number of reference objects,
which act as prototypes. Recognizing known objects
from novel viewpoints occurs by mathematically interpolating
between two or more prototypes (Broomhead & Lowe,
1988) to compute what a novel view should look like in
that region of the shape space. The predicted view is then
matched to the novel view. Thus, when a novel view is relatively
close in the shape space to two stored views, as in the
interpolated case, the novel view should be similar to the
predicted view and therefore relatively easy to recognize. In
contrast, in the extrapolated case the more distant learned
view will tend to reduce the similarity between the predicted
and novel views. This is because similarity (and ease of recognition)
is assumed to be an inverse function of the distance
between the novel view and each of the stored views that
are combined to make the predicted view (Shepard, 1968;
Edelman, 1999, p. 128). Together, these assumptions predict
that performance should be better on interpolated views
than on extrapolated views. Further, the view combination
mechanism is more robust than normalization because it can predict recognition performance for views of entirely novel
objects (Edelman, 1995).
The view combination mechanism is similar in many respects
to the notion of generalization in the animal learning
literature (e.g., Honig and Urcuioli, 1981). In particular,
generalization is commonly believed to occur via the combination
of excitatory and inhibitory activation gradients that
have formed around representations of positive (S+) and
negative (S-) stimulus values, respectively (Spence, 1937).
When more than one stimulus is positive, as is the case
when there are two training views, then a positive gradient
would be expected to form around the representation of each
S+ view. Equally, a negative gradient would be expected
to form around the representation of each S- view. Thus,
when a novel stimulus view is presented, it causes excitation
and inhibition according to how similar it is to the S+
and S- views that are represented, respectively; the sum of
that excitation and inhibition determines the response. If the
representations at the two training orientations overlap, then
novel interpolated views should receive generalization from
both training views, whereas novel extrapolated views might
receive generalization from only one training view. Thus,
the commonly held conceptualization of stimulus generalization
makes the same predictions as the view combination
approach for the difference in ease of recognition for interpolated
versus extrapolated views, so that view combination
is, in principle, a kind of generalization. Mathematically interpolating
between radial basis functions is one method of
implementing view combination (Edelman, 1999; Bülthoff
& Edelman, 1992). A radial basis function is a kind of neural
network in which the responses of neural units are Gaussians;
thus, there is a gradual decay in the response of the
units as the dissimilarity between the stimulus image and the
learned views increases.
In general, we believe the important similarities between
the view combination approach and stimulus generalization
outweigh any implementation differences. For example, the
notion that similarity is a function of the inverse distance
between a novel view and a set of stored prototypes (or S+
representations) is equally functional in both schemes. Similarly,
differently tuned generalization gradients should act
in most circumstances like the differently tuned radial basis
functions (Edelman, 1999) that underlie viewpoint interpolation.
Importantly, both view combination and generalization
mechanisms provide a meaningful contrast to recognition by
normalization to a nearest neighbor.
It should be emphasized that both normalization and view
combination accounts of object recognition take a “viewbased”
approach to recognition. The primary differences between
them involve the way that shapes are represented and
the particular mechanism used to compare the learned views
with novel views. The growing evidence for human object recognition favors the view combination approach. As we
will describe below, the evidence for pigeons appears to be
following the same trajectory, but with some interesting and
subtle differences.
Studies on Viewpoint Dependence
Like humans, many animal species are constantly faced
with the problem of recognizing objects from varying perspectives
because our view of an object can change due to
movement of ourselves or the object. For ground-dwelling
animals, including humans, the most frequent type of change
in view is produced when an object is rotated in depth, or
equivalently, if we move in a circle around the object. Such
depth rotation can result in drastic changes to the 2D shape
of the object and to the object features that are visible. Intuitively,
some views should be easier to recognize than others
(e.g., a side-view vs. a front-view). For a flying animal, such
as a bird, multiple changes in viewpoint would be common
and would include changes from top to side views. How do
birds recognize as objects when seen from different views
and are the processes used similar to our own?
In the past few decades, researchers have begun to investigate
pigeons’ ability to recognize objects across changes
in view. Most studies of pigeons’ recognition of 3-D objects
rotated in depth have found that pigeons’ recognition is
view-dependent. For example, some studies show that when
pigeons are trained with a particular view of an object, they
may not be able to recognize (transfer) to novel views of the
same object (e.g., Cerella, 1977, 1990). Other studies have
found that pigeons could transfer to novel views of familiar
objects, but that they did not show rotational invariance
for novel objects (Watanabe, 1997). Still other studies have found systematic decreases in discriminative performance as
a function of rotation from the training orientation (e.g., Jitsumori
& Makino, 2004; Lumsden, 1970; Wasserman et al.,
1996). Wasserman et al. (1996) found that pigeons’ generalization
to novel depth rotations of line drawings of 3-D objects
increased substantially if they were trained with three
rather than one view of the objects. Overall, most research
to date suggests that pigeons’ recognition performance is
best described in terms of view-based processes. However,
systematic investigation of factors that influence object recognition
in pigeons and studies aimed at elucidating object
recognition processes have only just begun.
A. Effect of Distinctive Parts

Figure 2. Discriminative objects used for pigeons in the same-parts and different-parts groups. From Spetch, Kelly, and
Reid (1999). |
|

Figure 3. Discrimination accuracy as a function of rotation
from the training views for pigeons in the Same-Parts and
Different-Parts groups. The dashed line indicates chance
level. From Spetch, Kelly, and Reid (1999). |
|
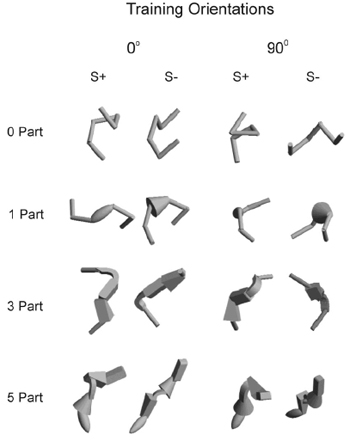
Figure 4. Objects used in each object-part condition. From
Spetch et al., 2001. |
|
Spetch, Kelly, and Reid (1999) conducted a preliminary
investigation of whether the presence of diagnostic features
would allow pigeons to generate viewpoint invariant object
representations. They trained pigeons to discriminate between
objects composed of red Lego pieces seen as digitized
images on a computer screen (Figure 2). For birds in
a Same-Parts group, the positive object (S+) differed from
three negative objects (S-) only in the arrangement of their
parts. For birds in a Different-Parts group, the positive object
was made of differently-shaped parts than the negative
objects and thus contained parts that could serve as diagnostic
features.
The pigeons saw the objects at six orientations in training
and then were tested at six novel orientations. Birds in the
Different-Parts group performed more accurately on both
training and test trials than birds in the Same-Parts group,
but importantly, the reduction in accuracy for the novel orientations
did not differ between the two groups (Figure 3). Thus, the presence of uniquely-shaped parts that could serve
as distinctive features appeared to enhance pigeons’ ability
to learn the discrimination between the objects but, in contrast
to results typically found with humans, it did not reduce
their viewpoint dependence.
To provide a more systematic investigation of the role of
distinctive parts in object recognition, and to provide a direct
comparison between pigeons and humans, Spetch, Friedman,
and Reid (2001) conducted a series of experiments
modeled after those conducted by Tarr et al. (1997). They
used a simultaneous discrimination task, which can be given
to both humans and pigeons, and they tested both species under
the same four conditions used by Tarr et al., namely objects
composed of zero, one, three, or five distinctive geons
(Figure 4).
In each condition, pigeons and humans viewed an S+ and
an S- object, which were shown side by side on a computer
monitor (Figure 5), with position of the S+ varied randomly
across trials.
During training, pigeons were reinforced with food for
pecking on the S+ side and humans were reinforced with
points for selecting the S+ side with the corresponding arrow
key. The objects were shown in two views that were a rotational
distance of 90° apart from each other during training,
and they were shown in four novel orientations during subsequent unreinforced test trials. Consistent with the results
found by Tarr et al., humans showed much weaker viewpoint
dependence in the 1-part condition than in either the 0-part or multi-part conditions. Pigeons, however, showed strong
viewpoint dependence in all four conditions and thus did not
appear to benefit from the presence of a diagnostic feature in
the 1-part condition (Figure 6).
Together, these results suggested that the presence of a distinctive
geon that can serve as a diagnostic feature does not
produce a representation in pigeons that is viewpoint invariant.
This appears to be one difference between object recognition
processes in pigeons and humans. It is important
to note, however, that this does not mean that pigeons are
insensitive to the presence of diagnostic features, because in
the Spetch et al. (1999) study, diagnostic features facilitated
pigeons’ discrimination between objects even though it did
not facilitate their ability to recognize new views (i.e., to
generalize across views). One possible explanation for these
results rests on the fact that our diagnostic features were
based on three-dimensional shape cues alone. Although the
distinctive part facilitated their discrimination between the
objects at the training views, pigeons might not benefit from
the distinctive part if their representation of the part itself is
viewpoint dependent. The finding by Peissig et al. (2000)
– that pigeons show viewpoint dependence even for single
geons – is consistent with this possibility.
Figure 5. Human and pigeon viewing objects on the computer screen. From Spetch et al., 2001. |
|

Figure 6. Accuracy of pigeons and humans on tests at the
training orientation or at novel orientations for each object-
part condition. The dashed line indicates chance level.
From Spetch et al., 2001. |
|
B. Effect of Degree of Rotation from Nearest Training View
Although the effect of distinctive parts on object recognition
highlighted a difference between pigeons and humans,
the effect of degree of rotation from the nearest trained view
highlighted a similarity between the species. Many studies
of human object recognition have found that speed and or
accuracy of recognition decreases systematically as a function
of how far the object is rotated from the nearest stored
view. As noted earlier, this result is directly predicted by
view-based theories, and can be accommodated by the other
theories as well under certain conditions. This effect of degree
of rotation has also been observed in pigeons, both with
real 3-D objects (Lumsden, 1970) and with line drawings
of 3-D objects (Wasserman et al., 1996). In both of these
studies, the objects contained distinctive parts. Our studies
found a similar result, both for objects with distinctive
parts and for objects without distinctive parts. Specifically,
Spetch et al. (1999) found a systematic decrease in accuracy
for pigeons as a function of rotation from the nearest training
view in both the Same-Parts group and the Different-
Parts group (see above Fig 3). Decreases in accuracy and
increases in latency as a function of rotation from the nearest
training view also occurred for both species in Spetch et al.
(2001). These effects can be seen in Figure 7, for which the
data were collapsed across stimulus conditions (0-part, 1-
part, 3-part, and 5-part).
Thus, at least under certain conditions, degree of rotation
from the nearest training view is an important determinant of
object recognition for both species.
C. Recognizing Interpolated versus
Extrapolated Novel Views
Although humans in Spetch et al. (2001) showed an overall
decrease in recognition as objects were rotated further
from the nearest trained view, their recognition depended not
just on degree of rotation but also on how the object was
rotated relative to the two training views. Because the training
views were 90° apart within a 360° rotation circle, novel rotations could fall within the shortest distance between the
views, which is considered to be an “interpolated” novel
view, or they could fall outside of this shortest distance,
which is considered to be an “extrapolated” novel view (see
Figure 8).
Spetch et al. (2001) found that, although humans showed
decreases in recognition for novel extrapolated views, they
showed complete rotational invariance in their recognition
of novel interpolated objects. That is, they recognized novel
interpolated views as quickly and accurately as the trained
views (Figure 9, top). This finding was consistent with previous
findings for human object recognition by Bülthoff and
Edelman (1992).
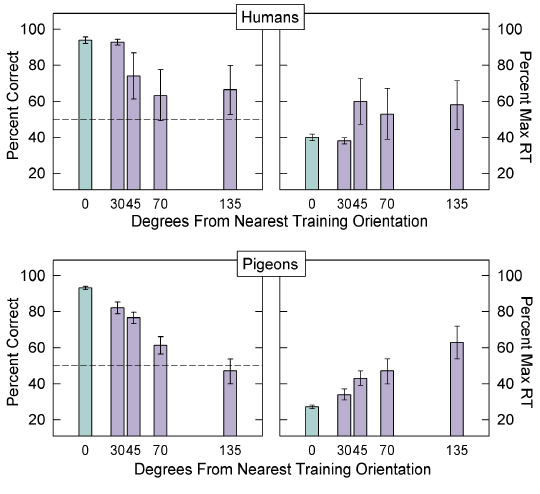
Figure 7. Accuracy and reaction time for pigeons and humans as a function of degree of rotation of objects from the nearest
training orientation. The dashed line indicates chance level. From Spetch, et al., 2001). |
|
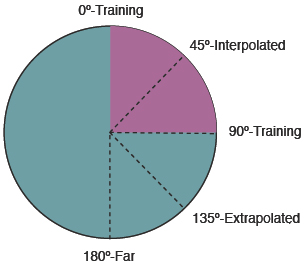
Figure 8. Diagram showing an example of the relationship
between two training views and an interpolated, extrapolated
and far novel view. From Friedman et al., 2005. |
|
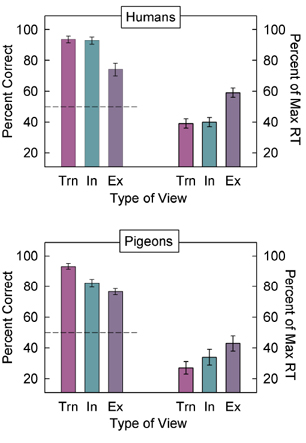
Figure 9. Accuracy and reaction time with training (Trn),
interpolated (In) and extrapolated (Ex) views for humans
and pigeons. The dashed line indicates chance level. From
Spetch et al., 2001. |
|
Interestingly, as seen in the bottom panel of Figure 9, pigeons,
unlike humans, did not show rotational invariance in
their recognition of interpolated novel views (Spetch et al.,
2001). However, in this initial study (and unlike the situation
depicted in Figure 8), the total amount of rotation differed
for interpolated and extrapolated novel views: In fact,
the interpolated novel views were closer in overall rotational
distance to the trained views than were the extrapolated
novel views. Consequently, we could not determine unambiguously
whether there was any advantage for the interpolated
views that was an effect of being interpolated per se.
Accordingly, we conducted another experiment to compare
interpolated versus extrapolated views with degree of rotation
equated (Spetch & Friedman, 2003). Both pigeons and
humans were trained with two views of 3-part objects. The
training views were 0° and 90° for one group, and 90° and
180° for a second group. Novel test views at 30° and 45°
rotations from each training view resulted in extrapolated
and interpolated novel views that were equated for degree of
rotation, and were counterbalanced for specific orientation across groups (see Figure 10).
A striking species difference emerged in recognition accuracy.
Humans showed a substantial decrease in accuracy and
increase in response time for the novel extrapolated views
compared to the trained views, but did not show a similar reduction in performance for the novel interpolated views
(Figure 11, top). By contrast, pigeons showed similar decreases
in accuracy for both interpolated and extrapolated
views (Figure 11, bottom). Thus, for pigeons, it appeared
to make little difference whether the objects were rotated
between the training views or outside of the shortest distance
between the training views.
The difference found with humans between interpolated
and extrapolated views has been taken as important evidence
for the appropriateness of the view combination approach as
a description of human object recognition (Bülthoff & Edelman,
1992; Edelman, 1999). As noted earlier, view combination
approaches permit some novel views to benefit from
their similarity to more than one learned view. The key factor
influencing recognition performance appears to be the
total rotational distance between the novel views and the
various training views; it is this distance that determines the
similarity between the novel test view and the stored views.
For example, in Spetch and Friedman (2003), the 30° interpolated
novel views were 30° from one training stimulus and
60° from the other, but the 30° extrapolated novel views were
30° from one training stimulus and 120° from the other (see
previous Figure 10). Thus, neither interpolated nor extrapolated
test views were equidistant to the training views, but
the interpolated views were closer on average to the training
views than were the extrapolated views. In this experimental
situation, the participants were 23% more accurate and 133
ms faster to respond to the 30° interpolated views than to
the 30° extrapolated views. Bülthoff and Edelman (1992) report
similar data over multiple interpolated and extrapolated
views that were both equidistant and non-equidistant to the
training views.
Notably, the original view-based approach predicts that
there should be similar decrements in performance to both
the interpolated and extrapolated views, and the view-invariant
approach predicts no decrements to either novel view.
Consequently, being able to demonstrate performance differences
between interpolated and extrapolated views is strong
evidence in support of the view combination mechanism.
Figure 10. Stimuli and design of training and test views used in Spetch & Friedman, 2003.
|
|

Figure 11. Accuracy and reaction time on tests with training
(Trn), interpolated (In), extrapolated (Ex) and far views
of the objects. The dashed line indicates chance level. From
Spetch & Friedman, 2003. |
|
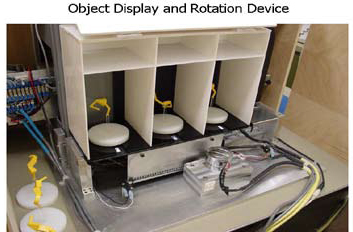
Figure 12. Back view of apparatus used to display and rotate
actual 3-D objects. See Friedman et al., 2003 for details. |
|
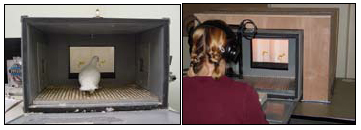
Figure 13. Front view of the object rotation apparatus as
used for pigeons and humans. From Friedman et al., 2003. |
|
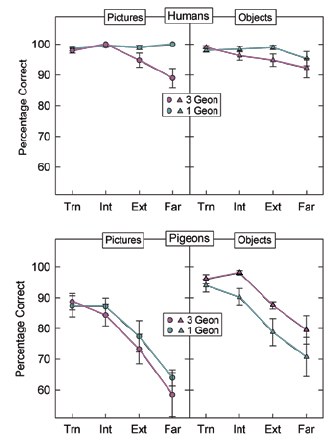
Figure 14. Percent correct for humans and pigeons as a
function of object type (1-geon or 3-geon), viewing condition
(pictures or directly viewed objects) and pose. Trn =
training, Int= interpolated, Ext= extrapolated. Chance
level accuracy is 50%. From Friedman et al., 2005. |
|
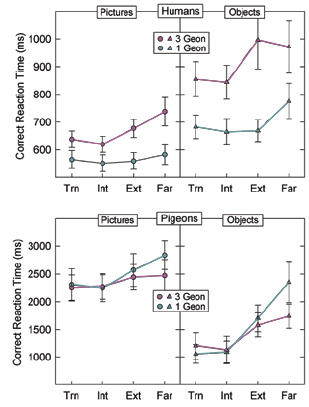
Figure 15. Reaction times for humans and pigeons as a function
of object type (1-geon or 3-geon), viewing condition
(pictures or directly viewed objects) and pose. Trn = training,
Int= interpolated, Ext= extrapolated. From Friedman
et al., 2005. |
|
D. Seeing the real thing: Pictures versus
direct views of objects
For practical reasons, almost all studies of object recognition,
both with humans and animals, present the objects as
images in slides or on a computer screen. This presentation
mode allows for rapid, automated presentation of objects in
various views. However, such a mode of presentation requires
not only object recognition processes, but also processes
for interpreting the images as representations of 3-D
objects. Moreover, although images of objects may provide
pictorial cues to depth, they do not provide other cues that
may be important for detecting depth information in the real world, such as binocular disparity and stereopsis. Therefore,
it is important to determine whether the processes identified
in studies of object recognition using pictures reflect the
processes used when recognizing objects viewed directly.
This consideration is particularly important for comparative
studies of object recognition. In particular, whereas adult humans
have extensive experience at interpreting pictures as
representations of actual 3-D objects, the same is not true
for pigeons. Thus, any differences that emerge between species
from studies using pictures could be due to differences
in picture interpretation processes instead of, or in addition
to, differences in object recognition processes. Therefore, it
was important to compare object recognition in pigeons and
humans using actual objects to determine whether the differences
we found in our previous studies were dependent on
the use of pictorial stimuli.
The first step in our endeavor to use actual objects was
to devise an apparatus that would allow automated presentations
of objects from multiple viewpoints. With the help
of our technician, Isaac Lank, we created an apparatus that
allowed us to present objects side by side, and to rapidly
and automatically rotate the objects between trials to present
them in any of 100 orientations (Figure 12).
The object tray contained three compartments for objects,
but only two were visible to the subject (Figure 13). By placing
two identical objects in the outside compartments, and
by sliding the tray randomly back and forth between trials,
we could thereby randomize the left-right location of the
positive object across trials. The apparatus was designed for
use with both pigeons and humans.
To create objects similar to those used in our previous studies
(Spetch et al., 2001; Spetch & Friedman, 2003), we used
a 3-D printer to make physical instantiations of the 1-part
and 3-part objects (see Friedman et al., 2003 for details). For direct-viewing conditions, we displayed these objects in
the object-rotation device. For picture-viewing conditions,
we took digital photos of the objects at each orientation as
they appeared from the viewing area of the object rotation
device, and we presented these on the computer screen.
The 3-D presentation apparatus and stimuli allowed us to
conduct a series of experiments to determine whether the
species differences we had identified previously would appear
both when the objects were seen in photographs and
when they were viewed directly (Friedman, Spetch, & Ferrey,
2005). First, a comparison of recognition accuracy for 1-
part and 3-part objects replicated the previous finding that the
presence of a single distinctive part substantially decreased
viewpoint dependence for humans but not for pigeons, both
when viewing actual objects and their photographs (Figure
14). Thus, this difference between species in the influence of
a distinctive part did not appear to reflect picture interpretation
processes. Second, however, a comparison of performance
with interpolated and extrapolated views of objects
yielded an interesting but more complicated set of species
differences. In Experiment 1 of Friedman et al. (2005), the
two training views were only 60° apart, which was a smaller
distance than we had used in the previous studies. The results
for the humans were the same for pictures and direct
viewing of 3-D objects: They recognized novel interpolated
views faster than novel extrapolated views (Figure 15).
The surprising finding in this first experiment was that the
pigeons also showed better recognition of novel interpolated
views than of novel extrapolated views, both when the objects
were viewed directly and when they were presented in photographs. However, and importantly, pigeons’ performance
overall was faster and more accurate when they were
directly viewing the objects than when they were viewing
pictures of them. Thus, we tentatively concluded that pigeons
process actual objects and their photographs differently.
Because the difference for pigeons between interpolated
and extrapolated views with photographs was inconsistent
with our previous results, we conducted a second experiment
in which the training views were 90° apart, which was
the rotational distance we had used previously. In this case,
pigeons showed an advantage for interpolated views only
when viewing the objects directly (Figure 16). To summarize, when viewing photographs with a 90° rotational difference
between the two training views, we replicated our
previous finding with pigeons viewing photographs: They
showed no difference in accuracy between interpolated and
extrapolated views. However, when pigeons viewed the objects
directly, or when they viewed photographs with training
views that were only 60° apart, pigeons, like humans,
were better able to recognize novel interpolated than novel
extrapolated views. Notably, when there was a significant
interpolated-extrapolated difference, it was larger for responses
to the actual objects than to their images for both
species. Together, the two experiments suggest that for pigeons,
viewing objects directly invokes different processes,
and may involve different representations, than viewing their photographs.
One interesting difference between the species was that
humans’ correct reaction times were longer with objects than
with pictures whereas pigeons’ correct reaction times were
shorter with objects than with pictures. The data are consistent
with the interpretation that real objects are easier for
pigeons to discrimination than pictures, whereas humans,
who have extensive experience with pictures, are as good or
better at quickly recognizing objects in pictures.
So, how do we make sense of this pattern of results? The
key finding across Experiments 1 and 2 was that the pigeon’s
recognition performance to novel interpolated views of actual
objects was different than their performance with the photographs
of those views, but only when the training views
were relatively far apart. In a view combination approach,
recognition of a novel view that is equivalent or even better
than recognition of a learned view should only occur if
the two (or more) representations that were used in the recognition
process were sufficiently similar to each other and
to the novel view that they would provide activation above
a “recognition threshold.” Thus, because pigeons’ successful
generalization (or view combination) occurred in the 90°
training condition for actual objects but not for their images,
it implies that the representations that resulted from seeing
actual objects were more broadly tuned than were the representations
that resulted from seeing photographs. A related
possibility is that, in the 90° training condition, the pigeons
represented the two training views as distinctly different objects
when they were presented as pictures but as the same
object when they were real. This could be because the pictures
required interpretative processes that pigeons do not
have. If two views are determined to be views of the same
object, that may contribute to the breadth of their representations,
or to an increase in the connections between representations,
and hence, to the ability to generalize to representations
“in between” the views that were trained (see Figure
17).
At this stage we can only conjecture about the kinds of
cues that actual objects afford but which are absent in their
photographs. For example, the depth cues derived from binocular
disparity and stereopsis (see Zeigler & Bischof, 1993)
may allow a broader representation of the 3-D properties of
the objects. Alternatively, movement of the bird could afford
slight variation in the experienced views of the objects during
training. A study with humans failed to show that head
movement improved generalization to novel extrapolated
views of real objects, but we cannot rule out the possibility
that movement could have contributed to the broader representation
for pigeons. Nevertheless, the data underline the
importance of using actual objects as stimuli in assessing and modelling human and animal object recognition.

Figure 16. Reaction times for pigeons as a function of viewing condition (pictures or directly viewed objects) and pose. Trn
= training, Int= interpolated, Ext= extrapolated. The dashed line indicates chance level. From Friedman et al., 2005. |
|
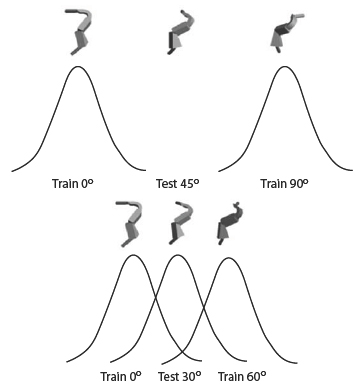
Figure 17. Diagram showing why generalization would facilitate
recognition of interpolated views when the training
views are close together (lower figure) but not when they are
far apart (top figure). With close training views, the recognition
functions for the two views overlap and hence an interpolated
view would benefit from combined generalization
from both views. |
|
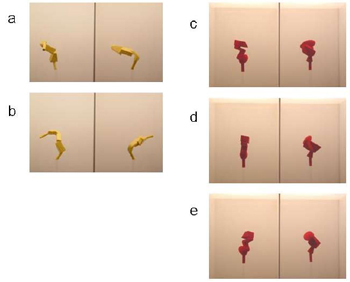
Figure 18. Stimuli used to assess transfer between directly viewed objects and their pictures. Objects and views a and b were used for some pigeons and objects and views c, d, and e were used for other pigeons. From Spetch & Friedman, submitted. |
|
Studies of transfer between pictures and objects
The extensive use of pictorial displays in comparative
studies of visual cognition not only raises questions about
their external validity with respect to real world objects, but
also raises the interesting question of whether non-humans
recognize the correspondence between the pictures and the
objects or scenes they represent. This question has been addressed
in numerous studies, some of which are nicely summarized
in a recent book (Fagot, 2000). Briefly, there have
been two main approaches to the correspondence question.
One approach has been to look for evidence that animals
respond to pictures with the same behaviors that are elicited
by real stimuli (e.g., aggression or courtship). In birds, this
approach has sometimes, but not always, yielded positive
results (see review by Fagot, Martin-Malivel & Depy, 2000).
However, natural behaviors are often elicited by specific features
of a whole stimulus (i.e., a “sign” stimulus such as a
patch of color) and can therefore be elicited by highly artificial
renditions of the real object (e.g., Tinbergen, 1951). Thus, observing an appropriate reaction to a picture of an
object does not necessarily imply that the organism sees the
picture as representing the real object. In addition, natural
behaviors that occur in response to a single stimulus feature
may be genetically “hard-wired,” so they may have little to
offer our understanding of whether animals understand the
correspondence between objects and their pictorial representations
more generally.
The second main approach has been to look for transfer
of learned behavior from pictures to the real objects or
scenes they represent and vice versa. For birds, the results
of such studies have again been mixed. For example, most
demonstrations that transfer has occurred have shown positive
transfer in one direction only (e.g., Cabe, 1976; Cole
& Honig, 1994). Moreover, interpretation of such results is
difficult in cases where the learned discrimination could be
based on differences between 2-D cues, such as color (see
Watanabe, 1997). In such cases, transfer of the discrimination
might be based on the presence of these simple 2-D features
and may not require any recognition that the pictures
correspond to the real stimuli.
Our object rotation device (Friedman et al., 2003) provided
an ideal opportunity to assess whether pigeons could
show transfer of a learned discrimination based on the 3-D
properties of objects. Accordingly, we trained pigeons to
discriminate between identically-colored 3-part objects that
were learned from two or three views (Spetch & Friedman,
submitted; see Figure 18).
Half of the pigeons were trained first with the objects
shown as images on the computer screen, and the remaining
pigeons were trained with the directly-viewed objects.
Following training, the birds were transferred to the other
media. For some birds the contingencies for the two objects
remained the same (i.e., the S+ object remained positive and
the S- object remained negative), but for other birds, the contingencies
were reversed. We found that birds transferred
with the same contingency showed higher accuracy on the
first 250 trials, and met an accuracy criterion significantly
faster than the birds transferred with the reversed contingency
(Figure 19). Importantly, positive transfer was seen
in both directions: from objects to their pictures and vice
versa. This is the first time such equivalent transfer between
the two types of media has been demonstrated so clearly. At the same time, the birds appeared to clearly notice the
difference between the objects displayed in pictures and directly-
viewed objects because accuracy on the transfer test
in the same contingency group started well below the 80%
criterion that was reached prior to transfer.

Figure 19. Discrimination accuracy during the first 250 trials
following transfer from pictures to directly viewed objects
(top) or from directly viewed objects to pictures (bottom) for
pigeons transferred with the same or reversed reinforcement
contingencies. The dashed line indicates chance level. From
Spetch & Friedman, submitted. |
|
It should be emphasized that our use of identically-colored
objects that were learned and had to be recognized from
more than one viewpoint made it very unlikely that the birds
used a simple 2-D cue, or a genetically-driven sign stimulus to discriminate between the positive and negative objects.
Hence, in contrast to some other demonstrations of transfer,
recognition of the objects in the new presentation format
could not be based on a simple cue like a distinguishing color.
We think these results provide the strongest evidence yet
that pigeons do see some correspondence between objects viewed directly and in pictures. Again, however, the circumstances
in which this bidirectional transfer occurred were
specific: Transfer occurred following training with more
than one view of an object. We conjecture that this kind of
training is more conducive to apprehending the object’s 3-D
structure. If this result is upheld, then it has strong implications
for avian models of the human visual system, at least
for object recognition.
Studies of the Role of Dynamic Cues in Object Recognition
Sometimes objects can be recognized not just by their
static properties, such as shape or color, but also by their
characteristic motion. Consider a grasshopper and a snake.
These creatures have a characteristic biological motion and
we can easily discriminate between them simply by the way
they move. Vuong and Tarr (2004) identified several ways
that dynamic information might play a role in object recognition,
including the possibility that a) motion may enhance
the detection of an object’s structure, and hence, the
recovery of shape information; b) motion provides multiple
views of the object’s shape, and thus affords the opportunity
for broadly tuned representations; c) motion may permit
meaningful edges to be found more readily, and enhance the
segmentation of a scene into discrete objects, or into foreground
and background, which is a likely precursor to object
recognition; d) motion may provide information about how
2-D image features change over time; and e) motion may
allow observers to anticipate future views of objects. Thus,
in the real world, object recognition may often make use of
dynamic information. We therefore need to consider the role
of motion cues to develop a more complete understanding of
the processes underlying object recognition.
Several recent studies have demonstrated that dynamic information
contributes to object recognition in humans (e.g.,
Liu & Cooper, 2003, Stone, 1998, Vuong and Tarr, 2004).
For example, Vuong and Tarr (2005) investigated people’s
ability to learn and recognize dynamically-presented artificial
objects within a 4-object identification task. Some of
the objects were decomposable (i.e., objects made of several
geons that can be easily decomposed into parts), whereas
other objects were amoeba-like objects that were hard to decompose (see
Video 1 and
Video 2).
Each object was shown in a particular direction of
motion. After learning the discriminations, participants were
required to name each of the objects as soon as they could
once the movie started. Reversing the direction of motion
for each object on subsequent tests was found to impair object
identification; however, this impairment occurred only
for objects that were difficult to discriminate, either because
they did not contain decomposable parts or because they
were degraded by “dynamic fog” (see
Video 3 and
Video 4).
The role of dynamic information in pigeons’ object recognition has been investigated in a few recent studies. Cook
and Katz (1999) trained pigeons to discriminate between a
cube and a pyramid that were sometimes presented as static
views taken at random orientations along a particular axis,
and at other times they were presented as dynamically rotating
along the axis. Transfer tests in which the object color
was changed or the axis of rotation was altered revealed better
performance with dynamic presentations than with static
presentations. However, changes to the direction of motion
along the trained axis did not alter discrimination performance.
Thus, dynamic information appeared to contribute to, but was not essential for, the object discrimination.
In a somewhat different approach to investigating pigeons’
sensitivity to dynamic cues, Cook, Shaw and Blaisdell
(2001) showed that pigeons could discriminate motion
paths in terms of their interaction with objects. In particular,
the pigeons learned to respond differentially according to
whether the motion path of the camera’s perspective moved
through an object or moved around an object. (Figure 20).
The birds showed significant transfer to new objects, and a
disruption in performance when the coherence of the motion
path was eliminated by randomly re-arranging the video frames, indicating that the discrimination was based on motion
cues.
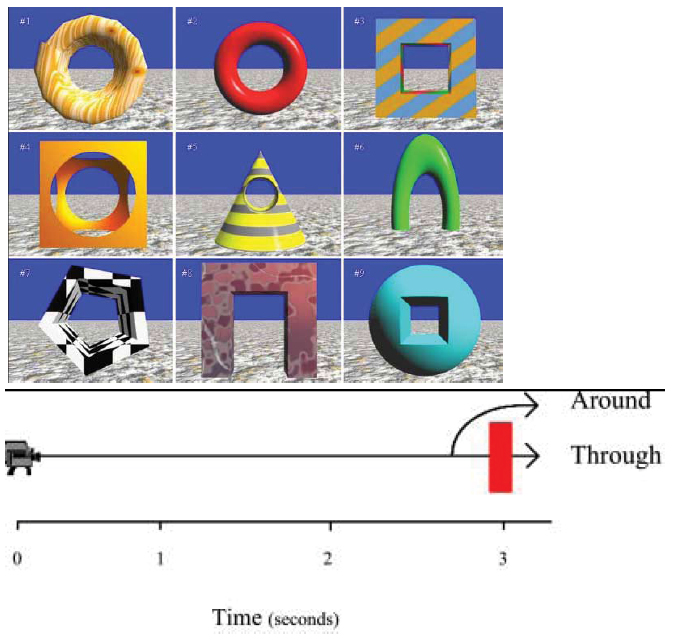
Figure 20. top: Objects used to create motion pathways (top) and diagram of two movement patterns in “Dynamic object
perception by pigeons: discrimination of action in video presentations” by Cook, Shaw and Blaisdell. Copyright, Springer-
Verlage, 2001, reprinted with permission. |
|
Evidence that pigeons are sensitive to biological motion
and can use natural motion cues to categorize stimuli was
provided by Dittrich, Lea, Barrett and Gurr (1998). Using a
discriminative autoshaping procedure, they found that some
pigeons could discriminate between full video scenes depicting
pigeons pecking and scenes depicting pigeons walking.
On transfer sessions in which the birds were tested with
point light displays of the movements alone, discrimination
was substantially lower than for full video displays, but was
significantly above chance level, suggesting that some discrimination
was based on motion cues alone. Diettrich et al.
also gave some pigeons discriminative autoshaping between
pecking and walking movements depicted with point-light
displays alone. Four of 12 birds learned the discrimination,
indicating that they could discriminate between these
behaviors on the basis of motion alone. However, none of
these birds showed significant transfer to full video displays.
Thus, their results suggest that pigeons are capable of discriminating
between biologically relevant motion cues, but there was considerable variability between birds in sensitivity
to these cues.
In a recent study, we compared pigeons and humans in
their discrimination of dynamically presented objects that
were each rotating in a particular trajectory (Spetch, Friedman
& Vuong, 2005). Both species were trained to respond
to one dynamic object (“Go” trials) and to withhold responding
to another object (“No Go” trials) that differed in 3-D
shape and rotated in the opposite direction along the same
trajectory. As in the Vuong and Tarr (2005) study, we used objects that humans
find easy to discriminate (objects that can be easily decomposed into parts; see
Video 5
and Video 6),
as well as objects that humans find hard to discriminate (amoeba-like objects
that are hard to decompose; see Figure 21,
Video 7, and
Video 8).
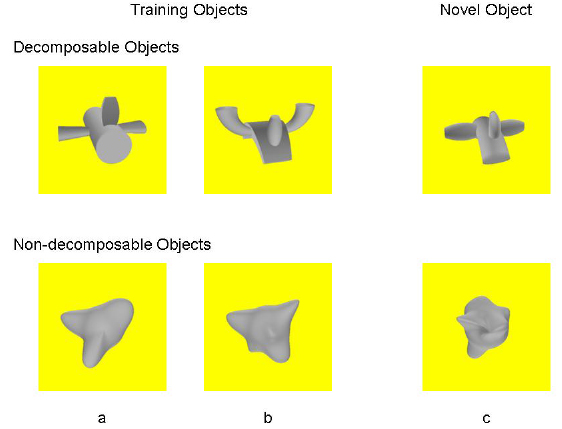
Figure 21.. Decomposable and non-decomposable object used in Spetch et al. (2005). Objects a and b were the training Go
and No Go objects (counterbalanced across participants) and objects c were the novel objects used in testing. |
|
In subsequent tests, we (a) presented the
learned objects in reversed direction of motion (see
Video 9,
10,
11,
and 12), which placed shape and motion
cues in conflict, (b) presented the learned objects in an entirely new
trajectory (see
Video 13, 14,
15, and
16), which tested for the effectiveness of shape cues alone, and (c)
presented a new object in the learned motions (see
Video 17,
18,
19
and 20), which tested for the
effectiveness of motion cues alone.
With decomposable objects, both pigeons and humans
showed a decrease in accuracy when the motion of the object
was reversed, or when a new motion was presented, but for
both species, the discrimination based on shape nevertheless
remained above chance level (Figure 22).

Figure 22. Proportion of Go responses to the Go and No Go stimulus by humans and pigeons in various test conditions.
Same = trained objects in their characteristic motions, Reversed = trained objects in the opposite motion, New Motion =
trained objects in an entirely new motion, New Objects = an entirely new object in the Go and No Go motions. The dashed
line indicates chance level. From Spetch et al., 2005. |
|
Further, humans showed this same pattern of results for
both decomposable and non-decomposable objects. In contrast,
pigeons but not humans, showed significant discrimination
of the new object based on learned motion cues alone.
That is, for both decomposable and non-decomposable objects
pigeons responded positively to the new objects when they appeared in the learned (S+) motion, and refrained from
responding when they appeared in the S- motion. In addition,
the pigeons, unlike the humans, showed no discrimination
between the learned non-decomposable objects when
they were presented in a new trajectory, and they responded
primarily on the basis of the motion rather than the shape
of the non-decomposable objects on the conflict test. It is
not clear whether this result occurred because the motion
overshadowed (i.e., interfered with learning about) the shape
cues of the objects, or whether the pigeons were unable to
recognize the invariance of the decomposable objects when
they moved in new ways. In either case, the pigeons discriminated between the objects primarily on the basis of
motion cues. Further research to determine whether motion
cues facilitate or interfere with recognition of static views
(e.g., Jitsumori & Makino, 2004) would be interesting to try
with our stimuli. Clearly, however, dynamic information can
contribute to object recognition in pigeons, and it does so
differently than in humans.
Summary
Our studies of object recognition in humans and pigeons
have revealed both similarities and differences between species
that have broadened our understanding of the processes
involved in ways that studying each species by itself could
not. The similarities between pigeons and humans include
the following. First, provided that objects do not contain
diagnostic features, both species typically show a systematic
decrease in recognition accuracy and/or speed as objects are
rotated in depth away from the nearest, single, trained view
(Spetch et al., 2001; Spetch & Friedman, 2003). This result
suggests that in general, object representations are viewpoint
dependent and that recognition depends on some sort of
view combination or generalization process. Second, object
recognition by both species is influenced by the nature of the
objects -- specifically whether they are decomposable into
parts and whether the parts are unique to each object (Friedman
et al., 2005; Spetch et al., 2001). Third, under some
circumstances, both species show better recognition of novel
views of objects that are interpolated between trained views
than of novel extrapolated views (Friedman et al., 2005;
Spetch & Friedman, 2003). Another way of describing this
effect is to say that both species can better recognize an object
from a novel view when the novel view is relatively
close to two trained views than when it is the same distance
away from only one trained view, or when the distance between
the novel view and the trained views is relatively far.
Finally, both species are sensitive to characteristic motion
cues associated with a dynamically viewed object, and show
reductions in recognition accuracy if the motion is altered
(Spetch et al., 2005). The extent of these similarities is impressive,
given that humans and pigeons differ in so many
ways, including evolutionary history and ecology, mode of
locomotion, and morphology of the visual system.
Within each of these areas of similarity, we also observed
some interesting differences in object recognition between
pigeons and humans. First, for humans, the systematic decrease
in object recognition as a function of depth rotation
is sometimes weak or does not occur if the objects contain a
single distinctive part that can serve as a diagnostic feature.
We did not see this for pigeons: They showed similar viewpoint
dependence whether the objects were composed of the
same or different parts (Spetch et al., 1999), and whether
paper-clip type objects had zero, one, or multiple added parts
(Spetch et al., 2001). Peissig, Young, Wasserman and Biederman
(2000) also found that pigeons’ recognition of single
geons generally decreased as the geons were rotated away
from the training view, a result that differs from some studies
with humans (Biederman & Gerharstein, 1993) but not others
(Tarr, Williams, Hayward, & Gautier, 1998). Thus, pigeons
show substantial viewpoint dependence even with objects
for which humans sometimes show viewpoint invariance.
This difference is not because pigeons are insensitive to the
nature of the objects. Pigeons show better discrimination of
objects that are composed of different parts than of objects
that are composed of the same parts (Spetch et al., 1999),
and they show greater control by the shape of decomposable
dynamic objects than by the shape of non-decomposable dynamic
objects (Spetch et al., 2005). Thus, the evidence to
date suggests that the structure of the objects affects pigeons’
discrimination between them, but not the viewpoint dependence
of their object representations.
A second difference between species is that, although pigeons
sometimes show better recognition of interpolated than
extrapolated novel views, they do so under a more restricted
set of conditions than we found with humans (Friedman et
al., 2005). Specifically, humans showed better recognition of
interpolated views under all conditions we tested, including
pictures or real objects and training views separated by 60°
or 90°. Pigeons showed the effect with real object when the
two training views were separated by either 60° or 90°, but
with pictures they showed the effect only when there was a
60° separation between the two training views. One interpretation
of this result is that pigeons form a more narrowly-
tuned representation of the objects seen in pictures than
objects seen directly, and in addition, that their representations
for objects seen in pictures are more narrowly tuned
that those of humans. As a result, when objects are seen in
pictures, the representations that pigeons form if the training
views are 90° apart may have insufficient overlap for there to
be a substantial benefit of interpolation. A related possibility
is that pigeons may encode such pictorial representations as
different objects.
A third difference we observed was that that pigeons appeared
to be more sensitive than humans to the characteristic
motion of a dynamically presented object (Spetch et
al., 2005). In particular, pigeons, but not humans, continued
to respond discriminatively when the trained motion directions
were carried by new objects. Thus, even in the case
of decomposable objects, for which pigeons showed greater
control by shape than by motion on conflict tests, they nevertheless
were able to recognize the characteristic motion
independently of the learned object shape.
Our results, which suggest that processes of object recognition
in pigeons may differ in interesting ways from
those in humans, complement other reports of differences
in visual cognition between pigeons and humans. For example, a number of studies, using various tests, have found
that pigeons fail to complete images of partially occluded
2-D objects (e.g., Fujita & Ushitani, 2005; Sekuler, Lee, & Shettleworth, 1996). Specifically, when the contour of one
object is occluded by another object, pigeons appear to see
the occluded object as a fragment rather than a whole object
that is behind another object. This finding stands in contrast
to results from humans and several other non-human species
(see Fujita, 2004). Kelly and Cook (2003) found that discrimination
of line orientation by pigeons and humans also
was affected in opposite ways by the addition of contextual
information. Specifically, humans’ discrimination between
oblique lines is enhanced by the addition of spatially contiguous
horizontal and vertical lines, (a configural superiority
effect) whereas pigeons showed reduced discrimination
under these conditions (see also Donis & Heinneman,
1993). Finally, Cavoto and Cook (2001) found that pigeons
responded more readily to the local information in a hierarchical
display. For example, when small letter O’s (local
information) made up a larger letter T (global information)
and vice versa, pigeons showed a local advantage in processing
the stimuli, which contrasts with the global advantage
more typically found with humans (Navon, 1977). Due to
the numerous differences in morphology, ecology and evolution,
it is not surprising that some differences in visual
cognition would emerge between humans and an avian species.
Perhaps more surprising are the findings of substantial
similarities between these species, both in object recognition
as reviewed here and in studies of other aspects of visual
cognition such as pattern recognition (e.g., Blough, 1985)
and texture discrimination (e.g., Cook, Cavoto, & Cavoto,
1996).
It is important to note that, as with the case of any differences
observed in comparative research, further research is
needed to determine their generality and causes. The possibility
that procedural and/or stimulus factors can influence
performance, and hence any differences observed, is
highlighted by our finding that pigeons responded more like
humans to interpolated views of objects when they viewed
them directly rather than in pictures. It will also be important
to assess the role of motion in object recognition using
directly-viewed objects. It is possible, for example, that the
shape of the object may become a more salient dimension
for pigeons when they view objects directly. If so, an interesting
question is whether shape would then overshadow
attention to the object’s characteristic motion and make the
performance of pigeons more like that seen for humans.
Directions for Future Research
Unlike investigations of other processes such as spatial
cognition (e.g., see Spetch & Kelly, in press; Cheng & Newcombe,
2005; Balda & Kamil, 2002) and memory processes
(e.g., see Grant & Kelly, 2002; Wright, Santiago, Sands,
Kendrick, & Cook, 1985), comparative research on the processes
underlying object recognition is in its infancy. The
research we have summarized provides a starting point, but
only a starting point, for how object recognition processes
compare across species. This work has revealed some interesting
similarities and differences between object recognition
processes in pigeons and humans. But much more work
with additional species, using different approaches, is needed
to make the study of object recognition a truly comparative
science.
Shettleworth (1993) nicely outlined two alternative research
programs within the field of comparative cognition,
namely an anthropocentric program and an ecological program.
She suggested that the anthropocentric program has
three essential features. First, as the name implies, it is human-
centered and asks whether non-humans can do what
humans can do. Second, it is concerned with demonstrating
whether animals can perform a particular task, rather than
understanding the conditions that encourage different cognitive
processes. Third, it assumes that evolution is a “ladder
of improvement” (p. 179). Shettleworth argues, and we believe
rightly so, that such an approach can miss some of the
richness of animal cognition and behavior. The alternative,
ecological program that Shettleworth advocates focuses on
the cognitive processes that animals use to solve ecologically
important problems, as well as why, and how, these
processes evolved. In this approach, selection of species
for comparison is thus based on evolutionary and ecological
considerations.
Although we would argue that the research we have summarized
does not follow the second two features of the anthropocentric
program, it is certainly human-centered in that
the comparisons were driven by what is known about object
recognition processes in humans and was concerned with
whether these processes are similar or different in the pigeon.
Both the experimental manipulations and the theoretical
ideas were derived primarily from research in human visual
cognition. However, we assumed that recognizing objects
is a generally important cognitive problem in most ecological
niches, much like “dealing with space, time and event
correlation” (Shettleworth, 1993, pg. 179), and hence some
generality in the processes involved in recognizing objects
is likely to be seen. What is clearly needed to complement
this work, however, is an ecological program of research on
object recognition. For example, it may be that specialized
object recognition processes have evolved that differ among
related species depending on their ecology (e.g., granivorous
versus predatory birds; nocturnal vs diurnal birds), primary
mode of locomotion, cues used for individual recognition
and mate selection, and so forth. Differences in the morphology
of sensory systems and in visual capabilities have
certainly evolved (Zeigler & Bischof, 1993), but comparisons between related species that differ in ecological factors
are needed to determine whether the underlying cognitive
processes are similarly specialized. For example, one might
predict that motion cues would be even more important for
predatory birds than for granivorous birds because predators
may use characteristic motion both to recognize the prey and
to anticipate the location of movement during capture (Kelly,
2002). However, many predatory birds, such as falcons, also
have extraordinary visual acuity. Hence, it would be interesting
to determine whether predatory birds would respond
more to shape or motion on a conflict test, and whether high
sensitivity to an object’s characteristic motion would facilitate
or overshadow their encoding of object shape.
A related interesting question for future research is to investigate
whether object recognition processes differ within
pigeons, depending on the specific visual system that is
used. As mentioned previously, pigeons have frontal fovea
for selecting grain and lateral fovea for more distant vision
and probably predator vigilance. The frontal visual field is
bilateral but the lateral visual field is monocular.
Because of the proximity of the objects in the operant
chambers we used for all the research we reviewed, the pigeons
presumably solved our tasks, both with pictures and
directly viewed objects, using the frontal visual system. It is
therefore of interest to assess object recognition in pigeons
for laterally-viewed distant objects. It is possible, for example,
that presence of a distinctive object part may facilitate
viewpoint invariant recognition when pigeons view distant
objects from the lateral field. One reason for making this
prediction is that we suspect that that viewpoint invariant
recognition might be particularly important when pigeons
view distant objects during flight because of the numerous
views in which the object can be seen. A second reason is that
recognition of objects from the lateral visual field, which is
monocular, would not benefit from binocular disparity, and
consequently might be more sensitive to diagnostic features
of objects. These ideas are pure speculation, however, and
need to be experimentally tested. It is possible that the different
properties of the frontal and lateral visual fields affect
low level vision only, and higher level cognitive processes
may not differ. For example, some principles of spatial cognition
in pigeons hold in both computer touch-screen tasks
and open-field tasks that require distant lateral vision (e.g., Spetch et al., 1996, 1997; see Cheng & Spetch, 1998 for a
review).
It may also be advantageous to consider the nature of the
stimuli and the visual systems employed when examining
other interesting findings related to object recognition in pigeons,
such as the failure to show perceptual completion and
the advantage for local stimuli in hierarchical displays. For
example, Fujita and Ushitani (2005) make the interesting
suggestion that pigeons’ lack of completion may be related
to their ecology: specifically, that their diet consists mainly
of small grains that would not need to be completed to be
recognized. In fact, they show that under certain circumstances,
not completing a occluded objects can facilitate object
detection, and they suggest that the lack of object completion
may sometimes be advantageous to pigeons. To our
knowledge, all tests of completion in pigeons have used pictorial
displays that would primarily activate the near frontal
visual system. Thus, it would be interesting to test whether
pigeons would show completion when viewing objects from
a distance, or when viewing real 3-D objects.
The last few decades have seen exciting developments in
the field of comparative cognition (for examples, see Cook,
2001 and abstracts from the Conference on Comparative
Cognition: http://www.comparativecognition.org ). Our understanding
of several important processes, such as memory
and spatial cognition has advanced remarkably, due to research
programs that are motivated by comparisons to human
cognition (e.g., Wright et al., 1985) as well as research
programs that are ecologically oriented (e.g., Balda & Kamil,
2002; Bond, Kamil, & Balda, 2003). We believe that these
alternative programs are complementary, and sometimes
can be merged in very interesting ways. A prime example of
such a merger is the investigation of “episodic-like memory”
in non-humans. The question itself comes from an anthropocentric
approach, but ecological considerations of situations
in which a non-human might need to remember what, when
and where have led to very interesting studies on this type
of memory process in a food-storing bird (e.g., Clayton,
Bussey, & Dickinson, 2003). Future research on object
recognition may also benefit from these complementary
approaches.
References
Balda, R. P., & Kamil, A.
C. (2002) Spatial and social cognition in corvids: An evolutionary
approach. In Bekoff, M, Allen, C. et al. (Eds.), The cognitive animal:
Empirical and theoretical perspectives on animal cognition (pp.
129-133). Cambridge, MA: MIT Press.
Biederman, I. (1987).
Recognition-by-components: A theory of human image understanding.
Psychological Review, 94, 115-147.
Biederman, I., &
Gerhardstein, P. C. (1993). Recognizing depth-rotated objects: Evidence
and conditions for threedimensional viewpoint invariance. Journal of
Experimental Psychology: Human Perception & Performance, 19,
1506-1514.
Blough, D. S. (1985).
Discrimination of letters and random dot patterns by pigeons and humans.
Journal of Experimental Psychology: Animal Behavior Processes. 11,
261-280.
Blough, P. M. (2001).
Cognitive strategies and foraging in pigeons. In R. G. Cook (Ed.),
Avian visual cognition [Online]. Available:
www.pigeon.psy.tufts.edu/avc/pblough/
Bond, A. B, Kamil, A. C.,
& Balda, R. P. (2003). Social complexity and transitive inference in
corvids. Animal Behaviour, 65, 479-487.
Broomhead, D. S., &
Lowe, D. (1988). Multivariable functional interpolation and adaptive
networks. Complex Systems, 2, 321-355.
Bülthoff, H. H., &
Edelman, S. (1992). Psychophysical support for a two-dimensional view
interpolation theory of object recognition. Proceedings of the
National Academy of Sciences, 89, 60-64.
Cabe, P. A. (1976).
Transfer of discrimination from solid objects to pictures by pigeons: A
test of theoretical models of pictorial perception. Perception and
Psychophysics, 19, 545-550.
Cavoto, K. K., & Cook,
R. G. (2001). Cognitive precedence for local information in hierarchical
stimulus processing by pigeons. Journal of Experimental Psychology:
Animal Behavior Processes, 27, 3-16.
Cerella, J. (1990).
Pigeon pattern perception: Limits on perspective invariance.
Perception. 19, 141-159.
Cerella, J. (1977).
Absence of perspective processing in the pigeon. Pattern Recognition,
9, 65-68.
Cheng, K., & Newcombe, N.
S. (2005). Is there a geometric module for spatial orientation? Squaring
theory and evidence. Psychonomic Bulletin & Review. 12, 1-23.
Cheng, K., & Spetch, M.
L. (1998). Mechanisms of landmark use in mammals and birds. In S. Healy
(Ed). Spatial representation in animals (pp. 1-17). Oxford: Oxford
University Press.
Clayton, N. S, Bussey,
T. J., & Dickinson, A. (2003). Can animals recall the past and plan for the
future? Nature Reviews Neuroscience, 4, 685-691.
Cole P. D., & Honig, W. K.
(1994). Transfer of a discrimination by pigeons (Columba livia)
between pictured locations and the represented environment. Journal
of Comparative Psychology, 108, 189-198.
Cook, R. G. (2001).
Avian visual cognition. [On-line]. Available:
www.pigeon.psy.tufts.edu/avc/
Cook, R. G., Cavoto, K.
K., & Cavoto, B. R. (1996). Mechanisms of multidimensional grouping,
fusion, and search in avian texture discrimination. Animal Learning &
Behavior, 24, 150-167.
Cook, R. G., & Katz, J. S. (1999). Dynamic object perception by pigeons. Journal of
Experimental Psychology: Animal Behavior Processes, 25, 194-210.
Cook, R. G., Shaw, R., & Blaisdell, A. P. (2001). Dynamic object perception by pigeons:
discrimination of action in video presentations. Animal Cognition, 4,
137-146.
Dittrich, W. H., Lea,
S. E. G., Barrett, J., & Gurr, P. R. (1998). Categorization of natural
movements by pigeons: Visual concept discrimination and biological
motion. Journal of the Experimental Analysis of Behavior, 70,
281-299.
Donis, F. J., &
Heinemann, E. G. (1993). The object-line inferiority effect in pigeons.
Perception & Psychophysics, 53, 117-122.
Edelman, S. (1999).
Representation and recognition in vision. Cambridge, MA: MIT Press.
Edelman, S. (1995).
Class similarity and viewpoint invariance in the recognition of 3D
objects. Biological Cybernetics, 72, 207-220.
Edelman, S., & Bülthoff,
H. H. (1992). Orientation dependence in the recognition of familiar and
novel views of three-dimensional objects. Vision Research, 32,
2385-2400.
Edelman, S., Bülthoff,
H. H., & Bülthoff, I. (1999). Effects of parametric manipulation of
inter-stimulus similarity on 3D object categorization. Spatial
Vision, 12, 107-123.
Fagot, J. (Ed). (2000).
Picture perception in animals. Philadelphia, PA, US: Psychology
Press/Taylor & Francis.
Fagot, J.
Martin-Malivel, J. & Depy, D. (2000). What is the evidence for an
equivalence between objects and pictures in birds and nonhuman primates?
In J. Fagot (Ed.). Picture Perception in Animals (pp. 295-320). Philadelphia,
PA: Psychology Press/ Taylor & Francis.
Friedman, A., Spetch,
M. L., & Ferrey, A. (2005). Recognition by humans and pigeons of novel
views of 3-D objects and their photographs. Journal of Experimental
Psychology: General, 134, 149-162.
Friedman, A., Spetch,
M. L., & Lank, I. (2003). An automated apparatus for presenting
depth-rotated three-dimensional objects for use in human and animal
object recognition research. Behavior Research Methods, Instruments,
and Computers, 35, 343-349.
Fujita, K. (2004). How
do nonhuman animals perceptually integrate figural fragments?
Japanese Psychological Research, 46, 154-169.
Fujita, K., & Ushitani,
T. (2005). Better living by not completing: A wonderful peculiarity of
pigeon vision? Behavioural Processes, 69, 59-66.
Grant, D. S., & Kelly,
R. (2001). Anticipation and short-term retention in pigeons. In R. G.
Cook (Ed.), Avian visual cognition [On-line]. Available: www.pigeon.psy.tufts.edu/avc/grant/
Honig, W. K., & Urcuioli,
P. J. (1981). The legacy of Guttman and Kalish (1956): 25 years of
research on stimulus generalization. Journal of the Experimental
Analysis of Behavior, 36, 405-445.
Husband, S., & Shimizu,
T. (2001). Evolution of the avian visual system. In R. G. Cook (Ed.),
Avian visual cognition [On-line]. Available:
www.pigeon.psy.tufts.edu/avc/husband/
Jitsumori, M., & Makino,
H. (2004). Recognition of static and dynamic images of depth-rotated
human faces by pigeons. Learning & Behavior, 32, 145-156.
Kelly, D. M. (2002).
Avian pattern and object perception. Dissertation Abstracts
International: Section B: The Sciences and Engineering, 63(6-B),
3050.
Kelly, D. M., & Cook,
R. G. (2003). Differential effects of visual context on pattern
discrimination by pigeons (Columba livia) and humans (Homo
sapiens). Journal of Comparative Psychology, 117, 200-208.
Kirkpatrick, K. (2001).
Object recognition. In R. G. Cook (Ed.), Avian visual cognition
[On-line]. Available:
www.pigeon.psy.tufts.edu/avc/kirkpatrick/
Logothetis, N. K., Pauls,
J., Bülthoff, H. H., & Poggio, T. (1994). View-dependent object
recognition by monkeys. Current Biology, 4, 401-414.
Liu, T., & Cooper L.A.
(2003). Explicit and implicit memory for rotating objects. Journal of
Experimental Psychology: Learning, Memory, and Cognition, 29,
554-562.
Lumsden, E. A. (1977).
Generalization of an operant response to photographs and
drawings/silhouettes of a three dimensional object at various
orientations. Bulletin of the
Psychonomic Society, 10,
405-407.
Metzler, J., & Shepard,
R. N. (1974). Transformational studies of the internal representation of
three-dimensional objects. In R.S. Solso (Ed.), Theories of cognitive
psychology: The Loyola Symposium. NY: Wiley.
Navon, G. (1977).
Forest before trees: The precedence of global features in visual
perception. Cognitive Psychology, 9, 353-383.
Peissig, J. J., Young,
M. E., Wasserman, E. A., & Biederman, I. (2000). The pigeon’s perception of
depth-rotated shapes.
In J. Fagot (Ed)
Picture perception in animals (pp. 37-70).
East Sussex: Psychology Press.
Poggio, T., & Edelman,
S. (1990). A network that learns to recognize three-dimensional objects.
Nature, 343, 263-267.
Sekuler, A. B., Lee.
J. A. J., & Shettleworth, S. J. (1996). Pigeons do not complete partially
occluded objects. Perception, 25, 1109-1120.
Shepard, D. (1968). A
two-dimensional interpolation function for irregularly spaced data.
Proceedings of the 23rd National Conference of the ACM,
517-524.
Shepard, R. N., &
Metzler, J. (1971). Mental rotation of three-dimensional objects.
Science, 171, 701-703.
Shettleworth, S. J.
(1993). Where is the comparative in comparative cognition? Alternative
research programs. Psychological Science, 4, 179-184.
Spence, K. W. (1937).
The differential response of animals to stimuli differing within a
single dimension. Psychological Review, 44, 430-444.
Spetch, M. L., &
Friedman, A. (2003). Recognizing rotated views of objects: Interpolation
versus generalization by humans and pigeons. Psychonomic Bulletin and
Review. 10, 135-140
Spetch, M. L., &
Friedman, A. (2005). Pigeons see correspondence between objects and
their pictures. Submitted for publication.
Spetch, M. L., Friedman,
A., & Reid, S. L. (2001). The effect of distinctive parts on recognition
of depth-rotated objects by pigeons (Columba livia) and humans.
Journal of Experimental
Psychology: General,
130,
238-255.
Spetch, M. L., Friedman,
A., & Vuong, Q. C. (2005) Dynamic Information Affects Object Recognition
in Pigeons and Humans. Submitted.
Spetch, M. L., & Kelly, D. M. (in press). Comparative spatial cognition: Processes in landmark
and surface-based place finding. In E. Wasserman and T. Zentall (Eds.),
Comparative Cognition: Experimental Explorations of Animal Intelligence.
Oxford: Oxford University Press.
Spetch, M. L., Kelly,
D. M., & Reid, S. (1999). Recognition of objects and spatial relations in
pictures across changes in viewpoint. Cahiers de Psychologie
Cognitive/ Current
Psychology of
Cognition, 18,
729-764.
Stone, J. V. (1998).
Object recognition using spatiotemporal signatures. Vision Research,
38, 947-951.
Tarr, M. J. (1995).
Rotating objects to recognize them: A case study on the role of
viewpoint dependency in the recognition of three-dimensional objects.
Psychonomic Bulletin and Review, 2, 55-82.
Tarr, M. J., & Bülthoff,
H. H. (1998). Image-based object recognition in man, monkey, and machine.
In M.J. Tarr & H.H. Bülthoff (Eds.), Object recognition in man,
monkey, and machine (pp. 1-20). Cambridge, MA: MIT Press.
Tarr, M. J., Bülthoff,
H. H., Zabinski, M., & Blanz, V. (1997). To what extent do unique parts
influence recognition across changes in viewpoint? Psychological
Science, 8, 282-289.
Tarr, M. J., & Gauthier,
I. (1998). Do viewpoint-dependent mechanisms generalize across members
of a class? Cognition, 67, 73-110.
Tarr, M. J., & Pinker,
S. (1989). Mental rotation and orientation dependence in shape
recognition. Cognitive Psychology, 21, 233-282.
Tarr, M. J., Williams,
P., Hayward, W. G., & Gauthier, I. (1998). Three-dimensional object
recognition is viewpoint dependent. Nature Neuroscience, 1,
275-277.
Tinbergen, N. (1951).
The study of instinct. Oxford: Oxford University Press.
Ullman, S. (1989).
Aligning pictorial descriptions: An approach to object recognition.
Cognition, 32, 193-254.
Vuong, Q. C., & Tarr, M.
J. (2004). Rotation direction affects object recognition. Vision
Research, 44, 1717-1730.
Vuong, Q. C., & Tarr, M.J.
(2005). Structural similarity and spatiotemporal noise effects on
learning dynamic novel objects. Perception, in press.
Wasserman, E. A.,
Gagliardi, J. L., Cook, B. R., Kirkpatrick-Steger, K., Astley, S. L., &
Biederman, I. (1996). The pigeon’s recognition of drawings of
depth-rotated stimuli. Journal of Experimental Psychology: Animal
Behavior Processes, 22, 205-221.
Watanabe, S.
(1997).Visual discrimination of real objects and pictures in pigeons.
Animal Learning & Behavior, 25, 185-192.
Wright, A. A. Santiago,
H. C., Sands, S. F., Kendrick, D. F., & Cook, R. G. (1985). Memory
processing of serial lists by pigeons, monkeys and people. Science, 2,
287-289.
Zeigler, H. P., & Bischof,
H. J. (1993). Vision, Brain, and Behavior in Birds.
Cambridge, MA: MIT Press. |
|